
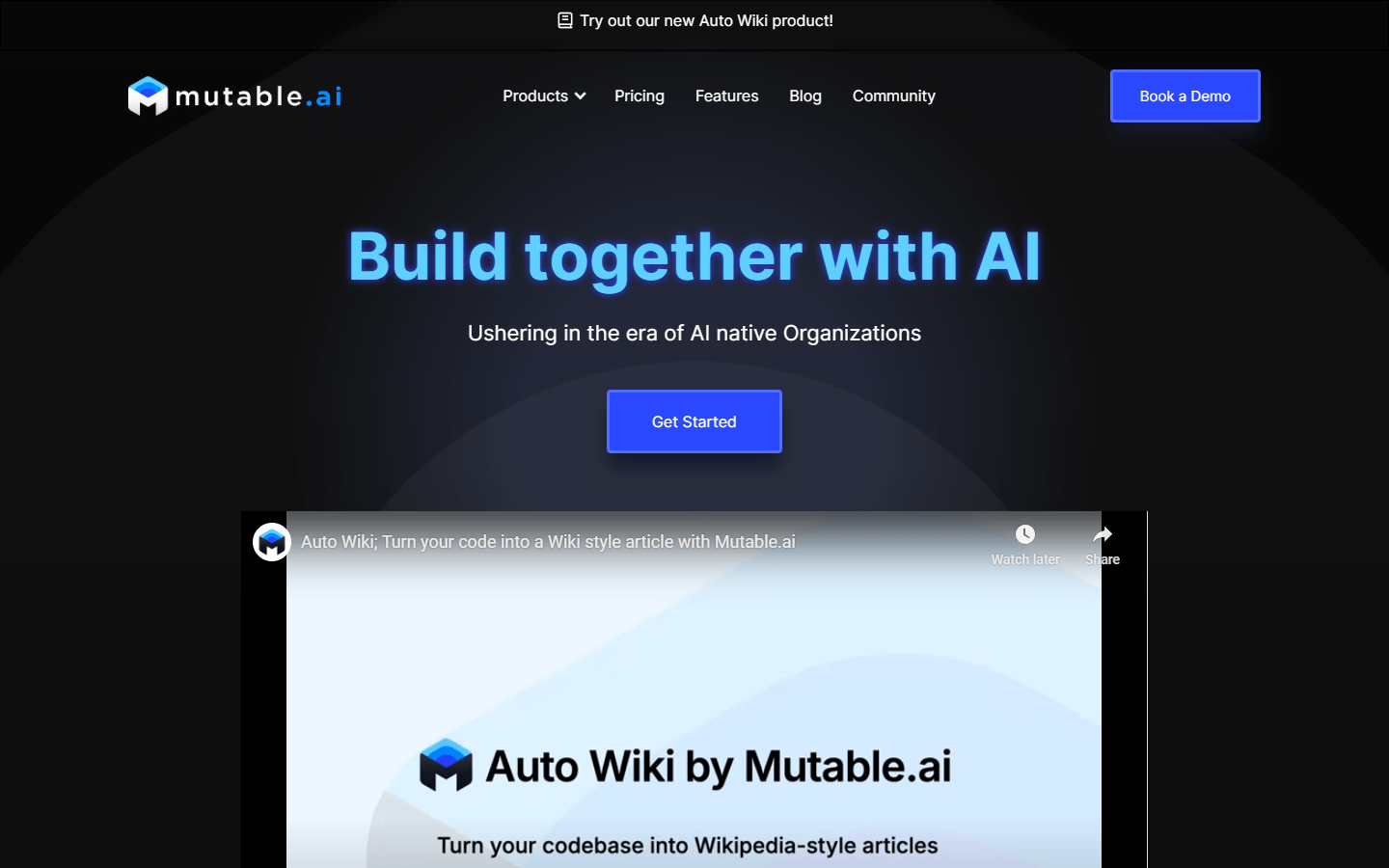
Overview
Mutable.ai is a company that develops AI-powered tools to help organizations improve their workflow and documentation. Their flagship product is an AI-generated wiki that can automatically convert code and other sources of information into easily digestible articles.
One of the key features of Auto Wiki is its ability to generate Wikipedia-style articles from code. This means that Auto Wiki can take your code and automatically create a well-structured and informative article that explains what the code does. This can be a huge time-saver for developers, as it eliminates the need to manually write documentation.
In addition to generating articles from code, Auto Wiki can also update documentation automatically when there is a change to the code. This means that your documentation will always be up-to-date, even if your code is constantly evolving.
Overall, Mutable.ai's Auto Wiki is a powerful tool that can help developers to improve their workflow and documentation. If you are looking for a way to automatically generate and update documentation, then Auto Wiki is definitely worth checking out.
Core Features
Automatically converts code into easy-to-understand, Wikipedia-style articles: This means that Auto Wiki can take your code and automatically create a well-structured and informative article that explains what the code does. This can be a huge time-saver for developers, as it eliminates the need to manually write documentation.
Automatically updates documentation when there is a change to the code: This means that your documentation will always be up-to-date, even if your code is constantly evolving.
Creates citations to the original source material: This ensures that your documentation is accurate and well-sourced.
Visually represents code: This can make it easier for people to understand complex code.
Use Cases
Individual Developer Workflow:
Accelerate personal project development: Quickly generate documentation as you code, reducing context switching and saving time.
Ensure code quality: Leverage AI suggestions to write clean, well-structured, and commented code that adheres to best practices.
Level up coding skills: Learn from the AI's insights and examples to improve your understanding of code patterns and algorithms.
Team Collaboration:
Streamline knowledge sharing: Create and maintain a centralized wiki of code documentation accessible to all team members.
Onboard new team members faster: Provide readily available documentation for existing codebases, speeding up integration.
Reduce communication overhead: Eliminate the need for manual documentation updates, fostering clearer understanding and smoother collaboration.
Software Product Documentation:
Generate user guides and technical manuals: Convert code logic into clear and concise explanations for users of varying technical expertise.
Maintain consistent API documentation: Automatically update API reference materials whenever code changes are implemented.
Improve customer support: Enable support teams to access readily available technical details for faster issue resolution.
Enterprise Knowledge Management:
Document internal systems and processes: Automate the creation of internal wikis, reducing reliance on manual documentation efforts.
Centralize knowledge bases: Facilitate easy access and sharing of critical information across teams and departments.
Improve knowledge transfer: Ensure smooth transitions between team members with readily available, up-to-date documentation.
Open-Source Project Development:
Maintain high-quality public documentation: Automatically generate accurate and consistent documentation for open-source projects.
Encourage community contributions: Improve project accessibility and attract developers with well-explained code logic.
Reduce maintenance burden: Minimize manual documentation updates while ensuring open-source knowledge remains readily available.
Research and Development:
Document experimental code and prototypes: Capture critical details and logic behind research projects for future reference.
Share research findings with collaborators: Generate clear and concise summaries of code functionality for dissemination.
Replicate research results: Facilitate future research by offering well-explained and documented codebases.
Technical Training and Education:
Create interactive coding tutorials: Automatically generate code explanations and exercises for students or trainees.
Develop self-paced learning materials: Make technical knowledge accessible through easily digestible and visual code representations.
Personalize learning experiences: Cater content to different learning styles by combining code explanations with visualizations and examples.
DevOps and Continuous Integration:
Automate documentation updates within CI/CD pipelines: Ensure documentation always reflects the latest code changes.
Improve traceability and auditability: Maintain a clear record of code evolution and changes implemented.
Streamline release management: Facilitate smooth releases with readily available and accurate documentation.
Security and Compliance:
Auto-generate security best practices documentation: Integrate security considerations into code documentation for improved awareness.
Maintain compliance documentation: Automatically update records to reflect changes in code that impact compliance requirements.
Improve security audits: Facilitate efficient audits by providing readily available and transparent documentation.
Data Science and Machine Learning:
Document data pipelines and models: Capture the logic and purpose behind data workflows and ML algorithms.
Improve reproducibility of research: Ensure experiments and results can be easily replicated and understood by others.
Facilitate knowledge sharing within data science teams: Foster collaboration and understanding by documenting complex data and ML processes.
Pros & Cons
Pros
Time-saving: Automate documentation, free up developer time for coding.
Accuracy: Generate consistent, factual docs based on code itself.
Efficiency: Update docs automatically upon code changes, reduce manual effort.
Accessibility: Create clear, Wikipedia-style articles for easy understanding.
Collaboration: Improve team knowledge sharing with centralized wiki system.
Onboarding: Accelerate new team member integration with readily available docs.
Knowledge Transfer: Ensure smooth transitions with up-to-date documentation.
Open Source: Encourage contributions with well-explained code logic.
Education: Develop interactive coding tutorials with explanations and exercises.
Security: Integrate security best practices into code documentation.
Cons
Limited understanding: AI cannot always grasp complex code nuances.
Initial setup: Requires integration and configuration for specific codebases.
Bias: AI models can inherit biases present in training data.
Error propagation: If code has errors, generated docs might misrepresent it.
Style variations: May require manual adjustments for consistent writing style.
Over-reliance: Could discourage developers from fully understanding code.
Cost: Paid service, might not be feasible for all projects or budgets.
Limited customization: Might not offer deep customization for specific needs.
Control: Developers cede some control over documentation presentation.
Security risks: Potential vulnerabilities if integration or access control is weak.
FAQs
mutable.ai Alternatives

GitMind
AI Powered Mind Map, Flowchart, Whiteboard.

Groq
A GroqLabs AI Language Interface.

What The Diff
The AI assistant for your pull requests

Zapier
Automate as fast as you can type

TensorFlow
An end-to-end platform for machine learning

Context Data
Data Processing & ETL infrastructure for Generative AI applications

v0.dev
Generate UI with simple text prompts. Copy, paste, ship.

MyShell
Democratizing & Decentralizing AI-native apps

Sora
Creating video from text

Alphadoc
Explain APIs and SDKs in a single environment.

Stepsize AI
Use GenAI to Report on Product Development

Amazon Bedrock
The easiest way to build and scale generative AI applications with foundation models

Supermaven
Free AI Code Completion

Mintlify
The documentation you want, effortlessly

Vercel AI SDK
The AI Toolkit for TypeScript

Hugging Face
The AI community building the future

ProtoUI
A concise summary of what your tool accomplishes.

Rely.io
The developer portal with an AI assistant you can speak with

AnotherWrapper
10+ customizable AI demo apps: pick one, make it yours, launch your startup quickly and start making money

Lazy AI
Platform to build full stack apps with prompts
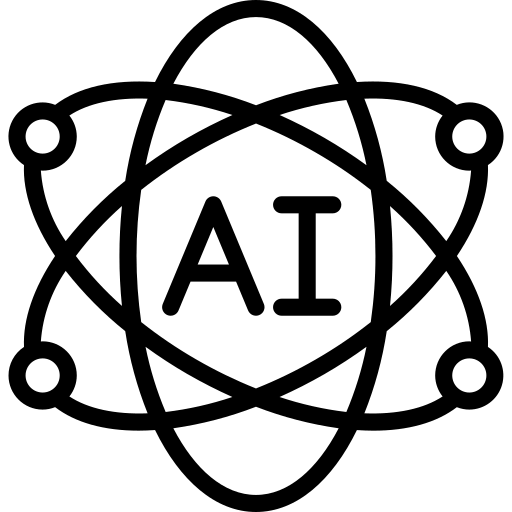
Webscrape AI
Automate Your Data Collection With No-Code

Phind
Get answers to complex questions with Phind's AI answer engine.

Tabnine
The AI coding assistant that you control

Supabase
Open Source Firebase Alternative

Bugasura
Issue Tracker for SaaS Teams, Partners & Customers

Accio
Query Your Data Warehouse Like Exploring One Big View

CodeDesign.ai
AI Website Builder Build & Host Your Site in Minutes!

SvelteLaunch
Svelte 5 Boilerplate for building AI apps quickly

Replicate
Run AI with an API

Blackbox AI
AI Code Generation, Code Chat, Code Search
![FLUX.1 [schnell]](https://cdn.brouseai.com/logo/gAATMVVzTB-BCugiC3hVp.png)
FLUX.1 [schnell]
The fastest image generation model tailored for local development and personal use

Durable
AI that builds a website for you.

Zerve AI
A Unified Developer Space For Data Science and AI

ChainGPT
Unleash The Power of Blockchain AI
![FLUX.1 [dev]](https://cdn.brouseai.com/logo/B6GQOp69ZOm-Zte81GQnv.png)
FLUX.1 [dev]
A 12 billion parameter rectified flow transformer capable of generating images from text descriptions

Cursor
The AI Code Editor

AutoGPT
The vision of the power of AI accessible to everyone

Lunary AI
The production platform for LLM apps.

Grit.io
Fix Technical Debt Automatically

Raycast
Your shortcut to everything

Claude 3.5 Sonnet
Our most capable model yet
![FLUX.1 [pro]](https://cdn.brouseai.com/logo/LjKDkTabCoBjQ9yqT998X.png)
FLUX.1 [pro]
State-of-the-art image generation with top of the line prompt following, visual quality, image detail and output diversity.

MindsDB
The middleware for building Custom AI

CodiumAI
Meaningful Code Tests for Busy Devs

Github Copilot
The world’s most widely adopted AI developer tool.
🚀 Build Your AI Startup in Hours!
10 customizable AI demo apps to help you build faster
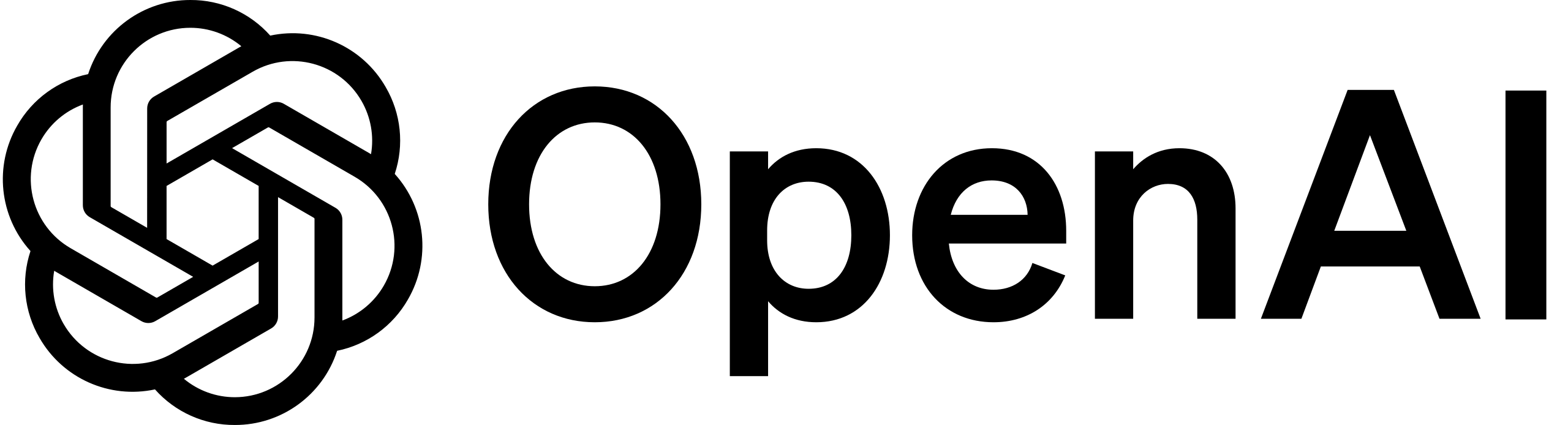
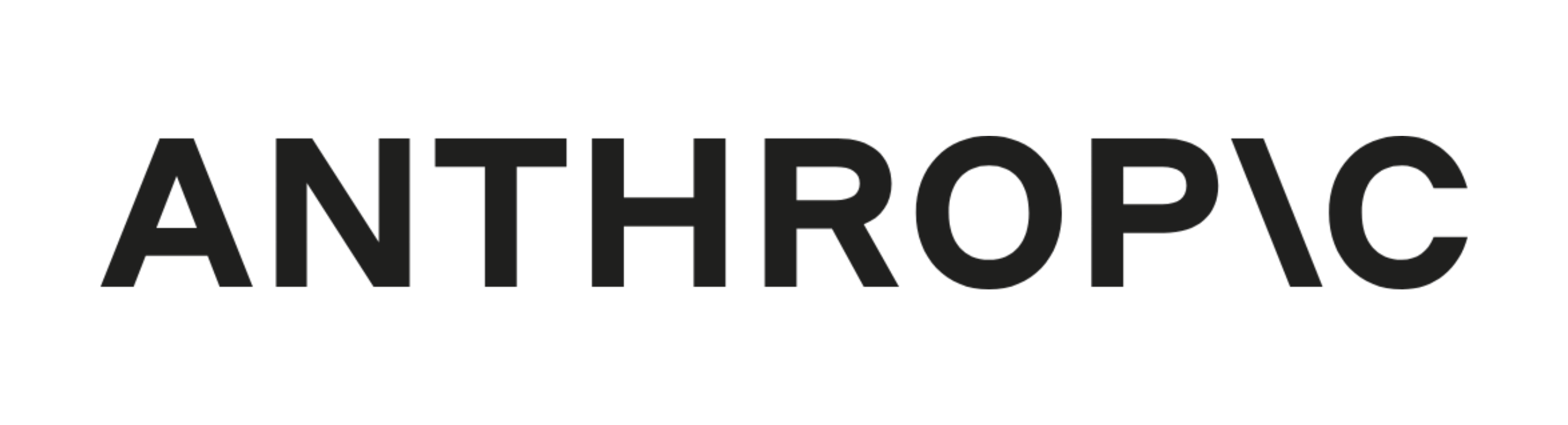
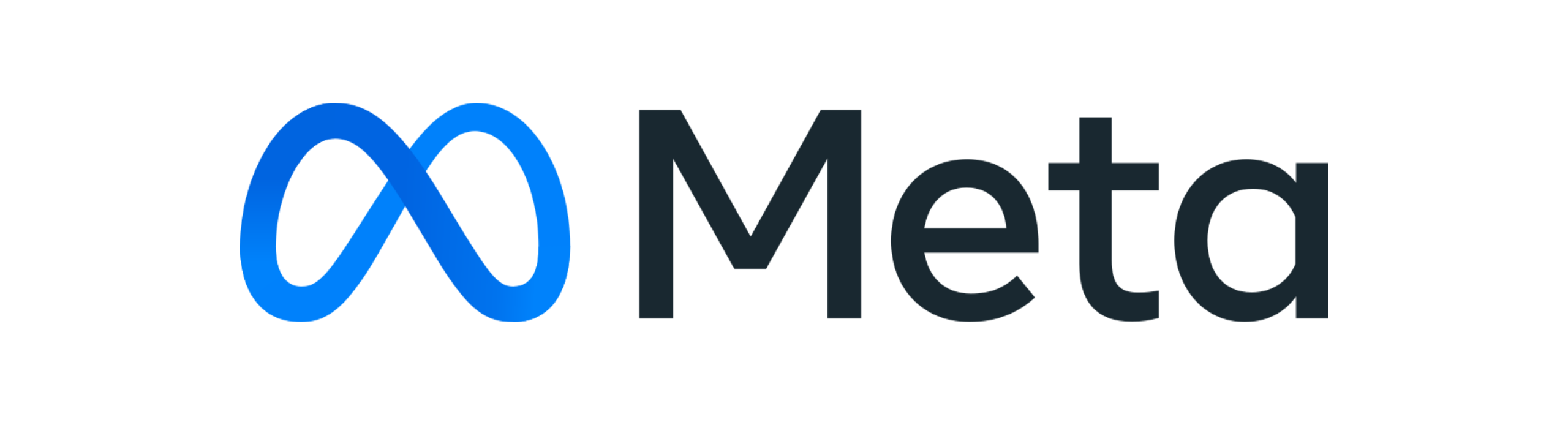
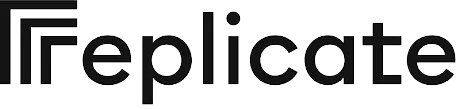
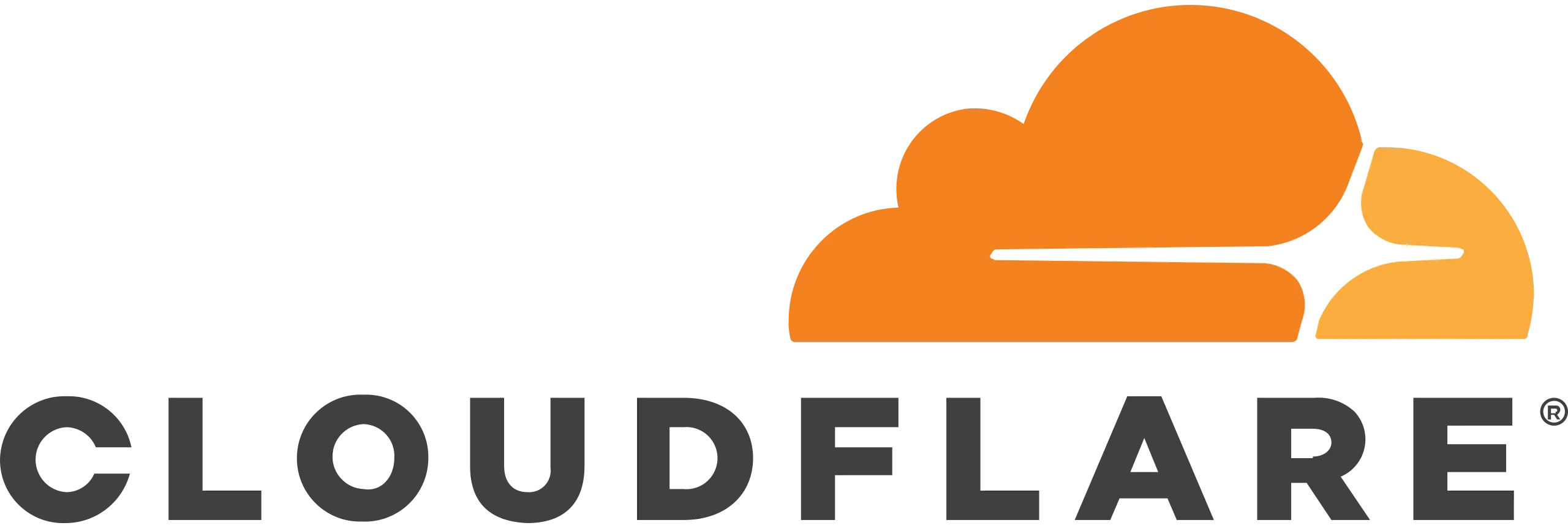
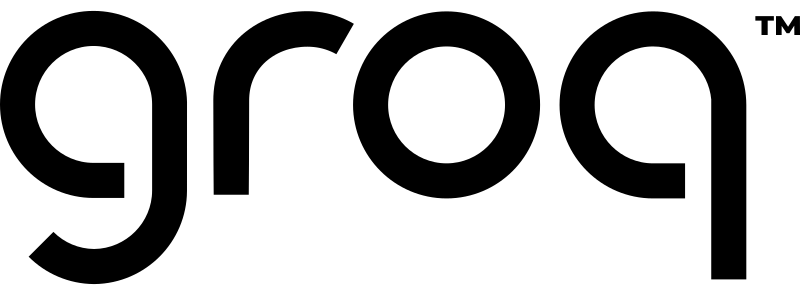
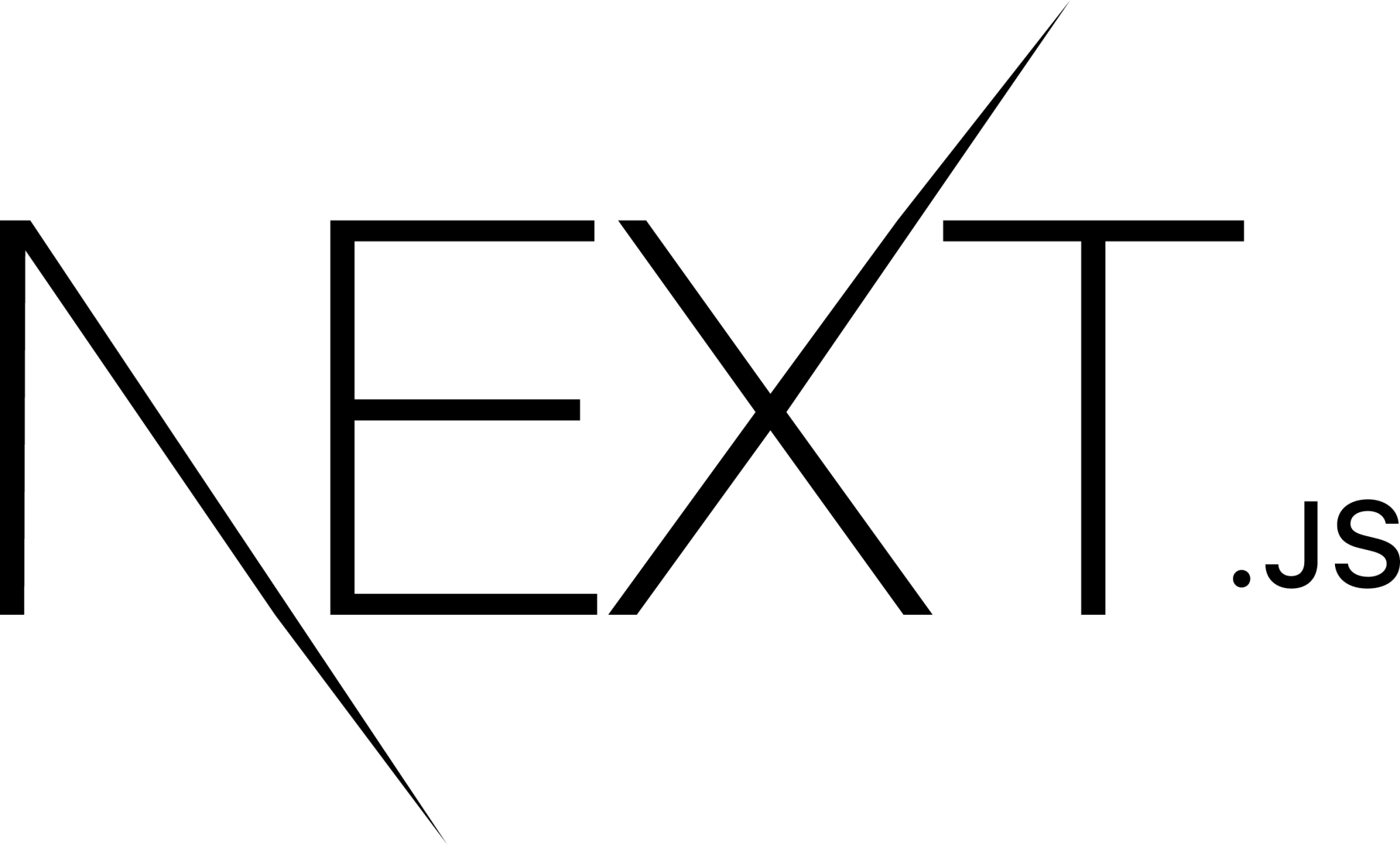

Chat with PDF
Build a PDF chatbot with vector embeddings and AI-powered Q&A
Text Generation
Generate structured content with GPT-4 and Claude 3
Image Generation
Create high-quality images with DALL·E and SDXL
And more
✨ Special offer: Get $100 off with code BLACKFRIDAY
Only 15 spots remaining at this price!
🚀 Launch Your Startup in Days, Not Weeks!
Supercharge your SaaS or AI tool development with ShipFast
Key Features:
NextJS Boilerplate
Production-ready setup with essential integrations
Payment Processing
Stripe & Lemon Squeezy integration
Authentication
Google OAuth & Magic Links for secure login
Databases
MongoDB & Supabase integration
Email Integration
Mailgun setup for transactional emails
UI Components
Ready-to-use components and animations
Time Saved:
- ✅ 4 hours on email setup
- ✅ 6 hours on landing page design
- ✅ 4 hours handling Stripe webhooks
- ✅ 2 hours on SEO tag implementation
- ✅ 3 hours on DNS record configuration
🎉 Limited Time Offer: $100 off for the next 12 visionaries! Only 12 spots left!
"I shipped in 6 days as a noob coder... This is awesome!" - Happy ShipFast User
"ShipFast helped me launch my AI tool and reach $450 MRR in just 10 days!" - Christian H.
Featured

SoundHound AI
Technology for a voice-enabled world
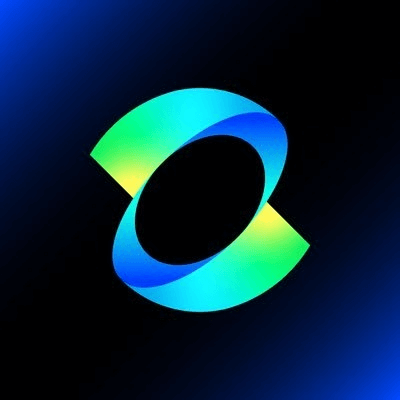
Kling AI
Next-Generation AI Creative Studio

QuillBot
QuillBot AI

Hugging Face
The AI community building the future
![FLUX.1 [pro]](https://cdn.brouseai.com/logo/LjKDkTabCoBjQ9yqT998X.png)
FLUX.1 [pro]
State-of-the-art image generation with top of the line prompt following, visual quality, image detail and output diversity.

Directonaut
Marketing accelerator as a software

VEED.IO
AI Video Editor - Fast, Online, Free

Movavi
AI-powered video editing tool

Runway
Tools for human imagination

Undetectable AI
AI Detector, AI Checker, & AI Humanizer

Luma AI
Dream Machine

AI Content Detector by Leap AI
Use our free AI Content detector to analyze text and see if it was generated by AI or not. AI Checker tool, 100% free forever.

Perplexity
Where knowledge begins

Luma AI by Serviceaide
Activate AI for your Enterprise

Stability AI
Activating humanity's potential through generative AI

Cursor
The AI Code Editor

Claude 3.5 Sonnet
Our most capable model yet

Easy Folders
All-in-one Chrome extension for ChatGPT & Claude.

Vercel AI SDK
The AI Toolkit for TypeScript

Vidnoz AI
Free AI Video Generator

Taskade
AI-Powered Productivity. A Second Brain for Teams

v0.dev
Generate UI with simple text prompts. Copy, paste, ship.

Lunary AI
The production platform for LLM apps.

Vheer
Free-to-use image generator

Gemini
Chat to supercharge your ideas - Google
![FLUX.1 [schnell]](https://cdn.brouseai.com/logo/gAATMVVzTB-BCugiC3hVp.png)
FLUX.1 [schnell]
The fastest image generation model tailored for local development and personal use
![FLUX.1 [dev]](https://cdn.brouseai.com/logo/B6GQOp69ZOm-Zte81GQnv.png)
FLUX.1 [dev]
A 12 billion parameter rectified flow transformer capable of generating images from text descriptions

Supermaven
Free AI Code Completion

Midday
Run your business smarter

AnotherWrapper
10+ customizable AI demo apps: pick one, make it yours, launch your startup quickly and start making money

Raycast
Your shortcut to everything

Capital Companion
Adding an AI Edge to Trading and Investing

Typefully
Write better content Grow your audience faster

Midjourney
Create AI generated images from a text prompt

ChatPDF
Chat with any PDF - Your PDF AI to ask your PDF anything

AI Paraphrasing Tool by Leap AI
Rephrase any text in seconds with this free AI paraphrasing tool. Rewrite, edit and change the tone of sentences with ease.

Groq
A GroqLabs AI Language Interface.