
Zerve AI
A Unified Developer Space For Data Science and AI
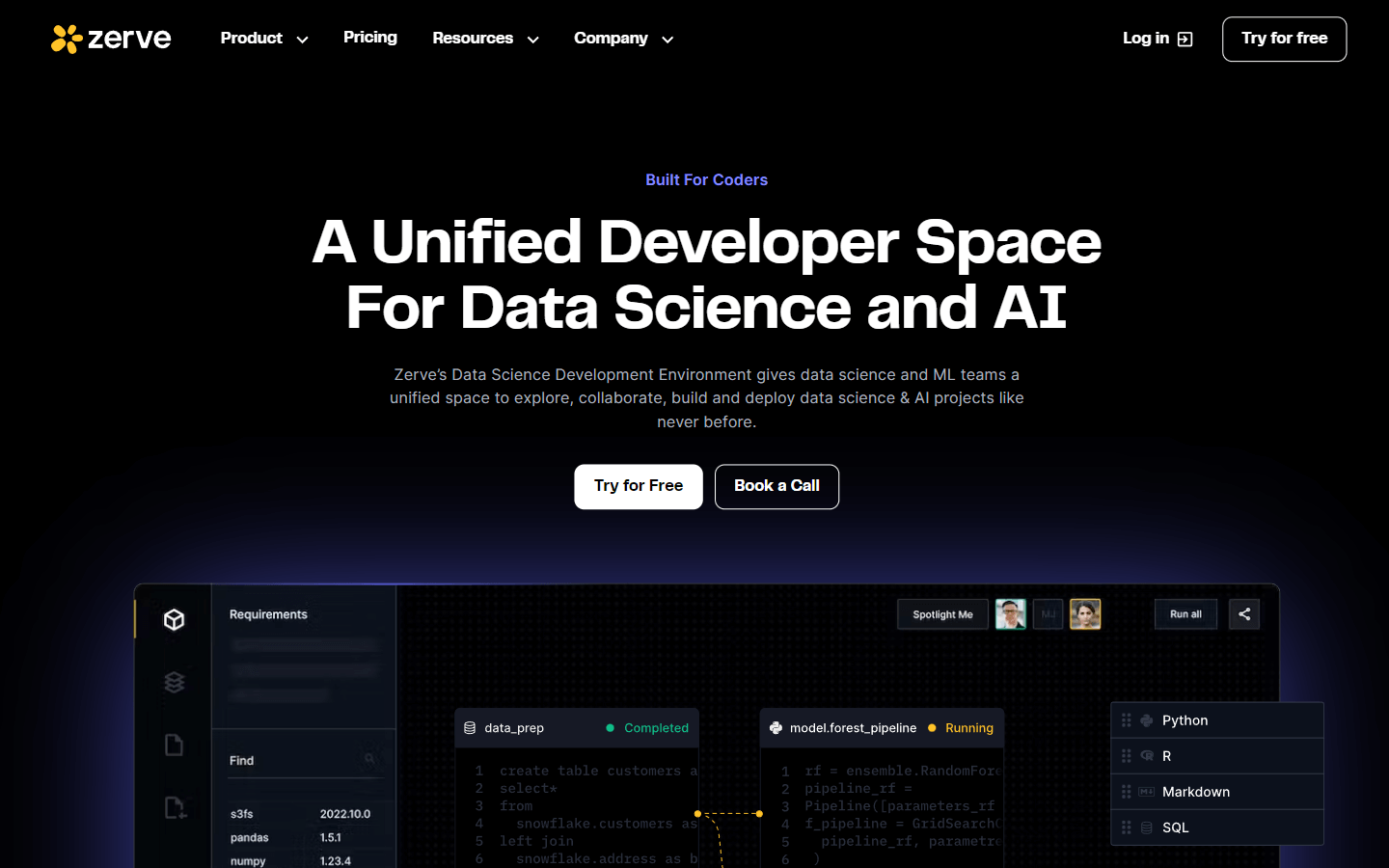
Overview
Zerve AI aims to revolutionize the field of data science and machine learning by offering a unified developer space specifically designed for these purposes. It functions as a single platform that manages the entire data science and AI project journey, from the initial exploratory data analysis (EDA) all the way through to deployment.
One of the key strengths of Zerve AI lies in its versatile environment, combining the best aspects of both notebooks and traditional integrated development environments (IDEs). This results in a user-friendly coding platform that leverages fully automated cloud infrastructure. This unique architecture means data science and ML teams can interactively explore data while being guaranteed consistent output every time. Users can seamlessly write and run code, add comments, review changes, and collaborate effectively, all within the same space.
Furthermore, Zerve AI fosters collaboration and efficiency through its innovative features. It boasts parallel processing capabilities, allowing team members to work on different aspects of the project simultaneously, even for tasks involving different languages like Python, R, and SQL. This significantly boosts productivity and streamlines the workflow. Additionally, compute optimization ensures efficient resource allocation, while notebook import enables effortless integration of existing work from other platforms.
Zerve AI goes beyond offering a mere coding environment. It incorporates essential tools for version control, dependency management, and organizational assets. This ensures project reproducibility, simplifies data governance, and empowers teams to share and reuse resources effectively. Moreover, the platform caters to enterprise needs with granular organizational controls and features like custom roles and advanced security protocols.
Overall, Zerve AI presents a compelling solution for data science and AI teams seeking to enhance their workflows, improve collaboration, and accelerate project delivery. Its unique blend of features, coupled with a user-friendly environment, positions it as a powerful tool capable of unlocking the full potential of data science initiatives.
If you'd like to delve deeper into specific aspects of Zerve AI, such as its pricing, integrations, or company background, feel free to ask!
Core Features
Parallel processing
Compute optimization
Notebook import
Language interoperability
Dependency management
Version control
Organizational assets
Org controls
Deployment
Integration with existing data stack
Use Cases
Financial fraud detection: A data science team uses Zerve AI to combine anomaly detection algorithms (Python) with visual exploration (Markdown) to uncover patterns in transaction data (SQL), flagging potential fraudulent activity in real-time.
Personalized product recommendations: A marketing team iterates on recommendation algorithms (R) within Zerve's collaborative environment, testing and refining predictions with parallel processing to optimize campaign performance.
Medical imaging analysis: A research team builds and shares deep learning models (Python) for analyzing medical images within Zerve, utilizing version control and organizational assets to ensure reproducibility and track research progress.
Customer churn prediction: A customer service team analyzes customer behavior data (SQL) using Zerve's language interoperability to develop churn prediction models (Python) and proactively intervene to retain valuable customers.
Predictive maintenance for industrial equipment: An engineering team deploys sensor data analysis models (Python) built in Zerve to predict equipment failures (using historical data and real-time monitoring), minimizing downtime and optimizing maintenance schedules.
Social media sentiment analysis: A brand management team uses Zerve to process and analyze social media data (using APIs) in real-time, gauging brand sentiment and identifying emerging trends with interactive visualizations.
Dynamic pricing for ecommerce: A pricing team leverages Zerve to develop and test pricing models (Python) based on factors like competitor analysis (web scraping) and demand forecasts, dynamically adjusting prices for optimal revenue.
Supply chain optimization: A logistics team analyzes historical and real-time data (various sources) within Zerve to optimize delivery routes and inventory levels, ensuring timely deliveries and minimizing costs.
Credit risk assessment: A financial services team builds and refines credit risk models (Python) within Zerve's secure environment, leveraging version control and audit trails to comply with regulations and ensure responsible lending practices.
Natural language processing for chatbots: A product development team utilizes Zerve's language interoperability to build and train chatbots (Python) with natural language processing capabilities, providing users with personalized and efficient support.
Pros & Cons
Pros
Unified Platform: Streamlines data science workflow from EDA to deployment.
Language Interoperability: Seamlessly combines Python, R, SQL, and Markdown.
Parallel Processing: Boosts team productivity with concurrent code execution.
Notebook Import: Integrates existing work from other platforms effortlessly.
Version Control: Ensures reproducibility and tracks project history effectively.
Collaboration Features: Facilitates seamless teamwork with shared workspace and comments.
Deployment Support: Simplifies model deployment to production environments.
Scalability: Adapts to growing data volumes and team size with ease.
Security: Offers robust security features for sensitive data and models.
Customization: Supports custom roles and integrations for tailored use cases.
Cons
Limited Free Tier: Free plan restrictions might hinder extensive exploration.
Learning Curve: New users might require time to adapt to the unique interface.
Vendor Lock-in: Switching platforms later might involve data migration challenges.
Limited Customization: Advanced users might desire deeper system customization.
Potential Cost Barrier: Enterprise pricing might be a consideration for smaller teams.
Limited Community Support: As a relatively new platform, the user community might be smaller.
Feature Gaps: Some specialized needs might require integration with external tools.
Integration Complexity: Integrating complex data pipelines might require technical expertise.
Long-Term Stability: As a young platform, long-term stability and development commitment remain to be seen.
Black Box Concerns: Understanding model internals might be challenging for certain use cases.
FAQs
Zerve AI Alternatives
π Build Your AI Startup in Hours!
10 customizable AI demo apps to help you build faster
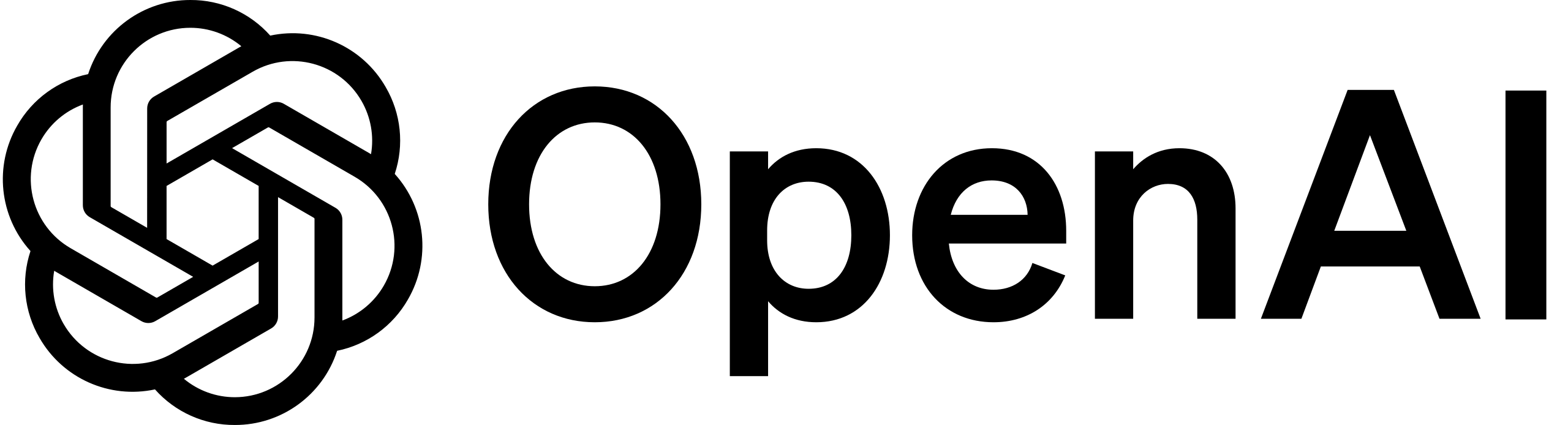
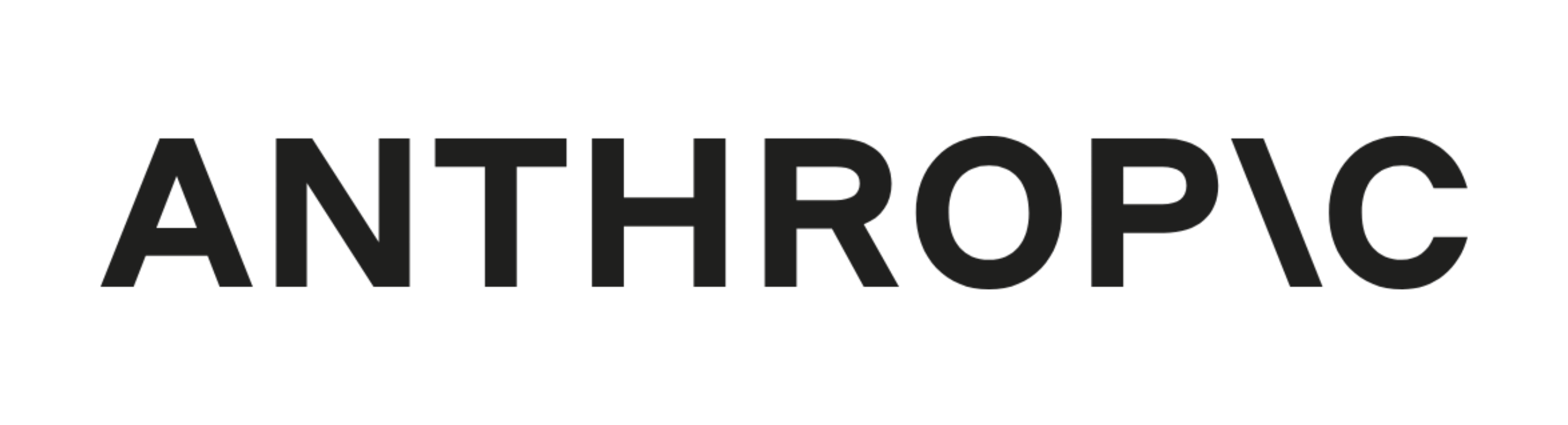
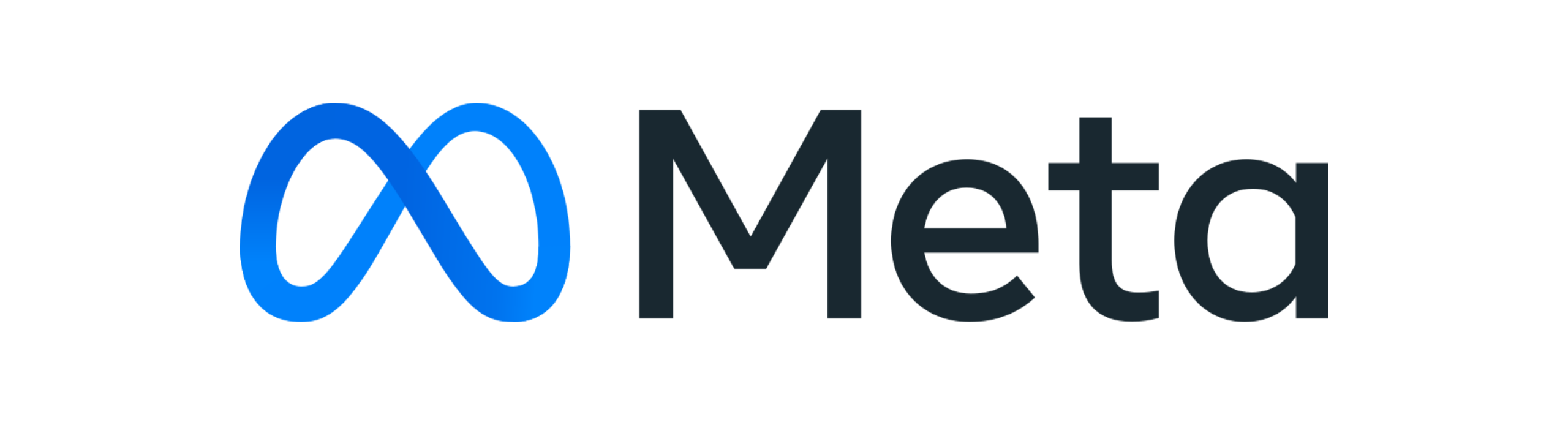
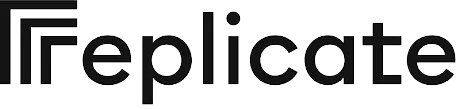
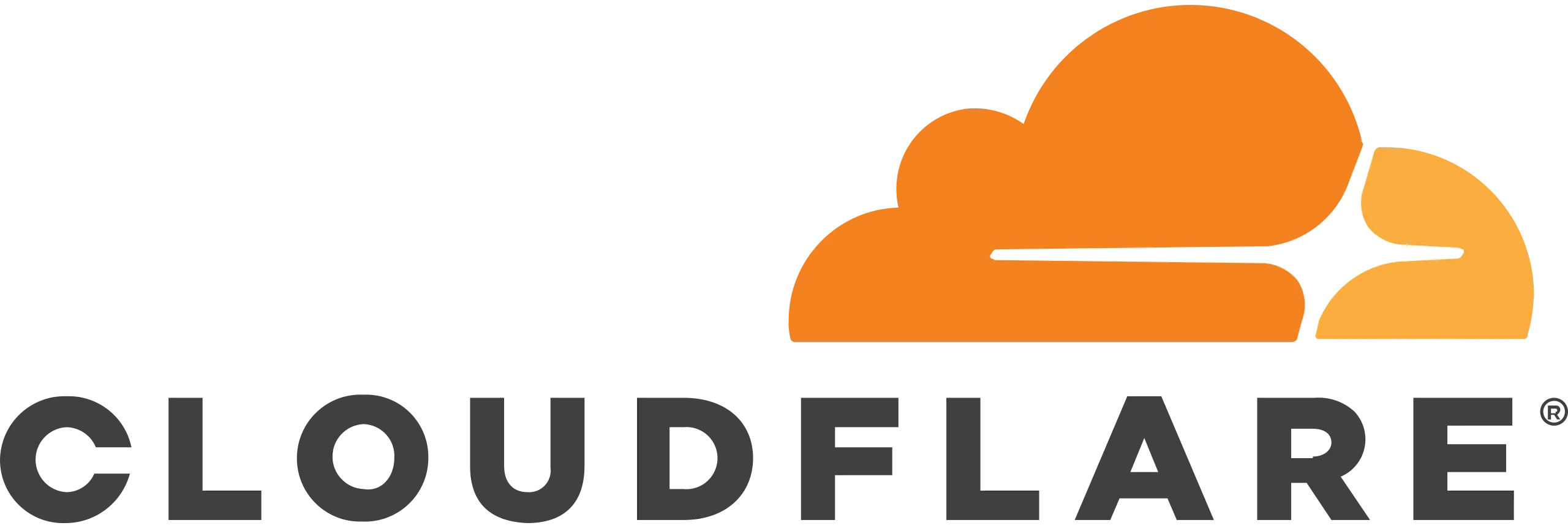
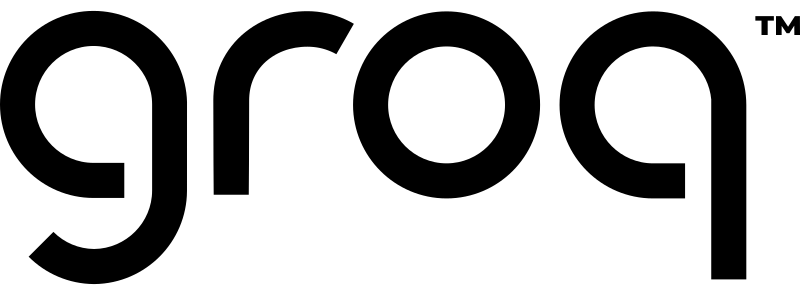
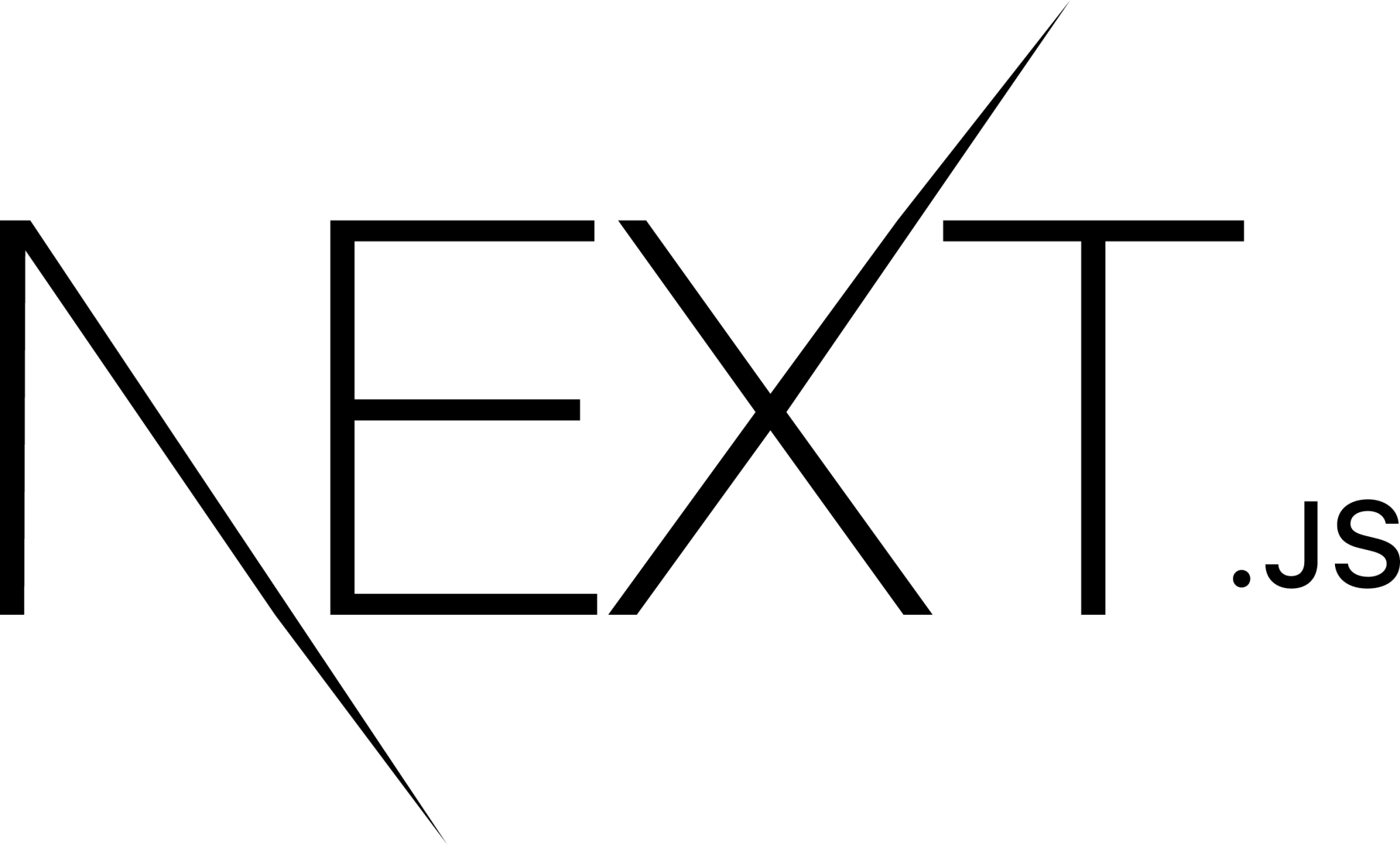

Chat with PDF
Build a PDF chatbot with vector embeddings and AI-powered Q&A
Text Generation
Generate structured content with GPT-4 and Claude 3
Image Generation
Create high-quality images with DALLΒ·E and SDXL
And more
β¨ Special offer: Get $100 off with code BLACKFRIDAY
Only 15 spots remaining at this price!
π Launch Your Startup in Days, Not Weeks!
Supercharge your SaaS or AI tool development with ShipFast
Key Features:
NextJS Boilerplate
Production-ready setup with essential integrations
Payment Processing
Stripe & Lemon Squeezy integration
Authentication
Google OAuth & Magic Links for secure login
Databases
MongoDB & Supabase integration
Email Integration
Mailgun setup for transactional emails
UI Components
Ready-to-use components and animations
Time Saved:
- β 4 hours on email setup
- β 6 hours on landing page design
- β 4 hours handling Stripe webhooks
- β 2 hours on SEO tag implementation
- β 3 hours on DNS record configuration
π Limited Time Offer: $100 off for the next 12 visionaries! Only 12 spots left!
"I shipped in 6 days as a noob coder... This is awesome!" - Happy ShipFast User
"ShipFast helped me launch my AI tool and reach $450 MRR in just 10 days!" - Christian H.
Featured

Vercel AI SDK
The AI Toolkit for TypeScript
![FLUX.1 [pro]](https://cdn.brouseai.com/logo/LjKDkTabCoBjQ9yqT998X.png)
FLUX.1 [pro]
State-of-the-art image generation with top of the line prompt following, visual quality, image detail and output diversity.

Gemini
Chat to supercharge your ideas - Google

ChatPDF
Chat with any PDF - Your PDF AI to ask your PDF anything

v0.dev
Generate UI with simple text prompts. Copy, paste, ship.

Groq
A GroqLabs AI Language Interface.

Supermaven
Free AI Code Completion

Typefully
Write better content Grow your audience faster

Easy Folders
All-in-one Chrome extension for ChatGPT & Claude.

AI Content Detector by Leap AI
Use our free AI Content detector to analyze text and see if it was generated by AI or not. AI Checker tool, 100% free forever.

Cursor
The AI Code Editor

Hugging Face
The AI community building the future

Raycast
Your shortcut to everything

Directonaut
Marketing accelerator as a software

AnotherWrapper
10+ customizable AI demo apps: pick one, make it yours, launch your startup quickly and start making money

Luma AI
Dream Machine

QuillBot
QuillBot AI

AI Paraphrasing Tool by Leap AI
Rephrase any text in seconds with this free AI paraphrasing tool. Rewrite, edit and change the tone of sentences with ease.

Lunary AI
The production platform for LLM apps.

Stability AI
Activating humanity's potential through generative AI
![FLUX.1 [dev]](https://cdn.brouseai.com/logo/B6GQOp69ZOm-Zte81GQnv.png)
FLUX.1 [dev]
A 12 billion parameter rectified flow transformer capable of generating images from text descriptions

Perplexity
Where knowledge begins

Luma AI by Serviceaide
Activate AI for your Enterprise

Midjourney
Create AI generated images from a text prompt

Vidnoz AI
Free AI Video Generator

Capital Companion
Adding an AI Edge to Trading and Investing

Movavi
AI-powered video editing tool

Undetectable AI
AI Detector, AI Checker, & AI Humanizer
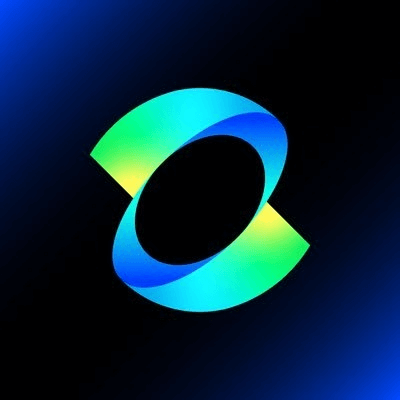
Kling AI
Next-Generation AI Creative Studio

Claude 3.5 Sonnet
Our most capable model yet

VEED.IO
AI Video Editor - Fast, Online, Free

Runway
Tools for human imagination

SoundHound AI
Technology for a voice-enabled world
![FLUX.1 [schnell]](https://cdn.brouseai.com/logo/gAATMVVzTB-BCugiC3hVp.png)
FLUX.1 [schnell]
The fastest image generation model tailored for local development and personal use

Midday
Run your business smarter

Taskade
AI-Powered Productivity. A Second Brain for Teams